Ever since ChatGPT’s release, AI has been a hot topic of discussion. People have gone from being amazed to being skeptical and everything in between. It seems a new controversy around AI comes up every day.
AI has revolutionized various industries, including healthcare, insurance, and banking. However, one of the critical challenges artificial intelligence faces is the phenomenon known as "AI hallucinations."
In this article, we'll explain what AI hallucinations are, why they occur, and how you can mitigate them. Our goal is to focus mainly on the needs of high-stakes, highly-regulated industries and the impact of hallucination on their usage of AI tools.
Key Takeaways
- AI hallucinations are a frequent issue in large language models (LLMs).
- AI hallucinations occur because of the model's inherent biases, lack of real-world understanding, or limitations in its training data.
- Produced content that is incorrect can be harmful and negatively affect decision-making processes.
- There are several ways companies and individuals can mitigate AI hallucinations.
What Is an “AI Hallucination”?
An AI hallucination occurs when a generative AI model creates false or misleading information and presents it as true. These errors can range from minor inaccuracies to completely fabricated details, which can be problematic. AI models can sometimes make these mistakes or hallucinations because they rely on statistical likelihoods rather than true comprehension.
It is very easy to get trapped in a back-and-forth with chatbots that leads to a dead end. We may think they are right because we can have human-like conversations with them. They give answers so confidently that people often forget that AI models can’t think and lack reason.
AI hallucinations can also happen in image recognition systems and AI image generators, but they are most common in AI text generators.
We can categorize AI hallucinations into three types:
- Factual errors
- Fabricated facts/information
- Harmful content
Examples of AI Hallucinations
There are many examples of AI hallucinations. Some of them might include:
- Generating fictional historical events or people.
- Providing inaccurate medical advice.
- Misinterpreting financial data, and many more.
As we will see in the next few examples, reporting feedback so tech companies can address hallucinations is important to ensure AI's reliability and accuracy.
Google’s Hallucination Examples
Google made its fair share of mistakes with its AI launches. In February 2023, in an effort to compete with Microsoft and OpenAI, Google introduced Bard, a chatbot, to challenge ChatGPT. They publicly announced Bard’s launch on X with the now-infamous James Webb Space Telescope gaffe.
Google’s Bard tweet features an animated graphic to demonstrate the user experience, where the AI incorrectly claims that the James Webb Space Telescope took the first-ever picture of an exoplanet. While the James Webb Telescope captured its first exoplanet image in September last year, the actual first picture of an exoplanet was taken in 2004.
The company's market value plummeted by $100 billion as a result.
Google had its second major fiasco with the Gemini launch a year later.
They received a major backlash after some users brought their screenshots results on X, exposing extreme racial imbalance. Users noticed that the system avoided creating images of white people and often produced inaccurate representations of historical figures.
Some of the images that drew criticism included a portrayal of four Swedish women, Founding Fathers, and portraits of a pope. Social media users criticized the company, accusing it of overreaching in its attempt to demonstrate racial diversity.
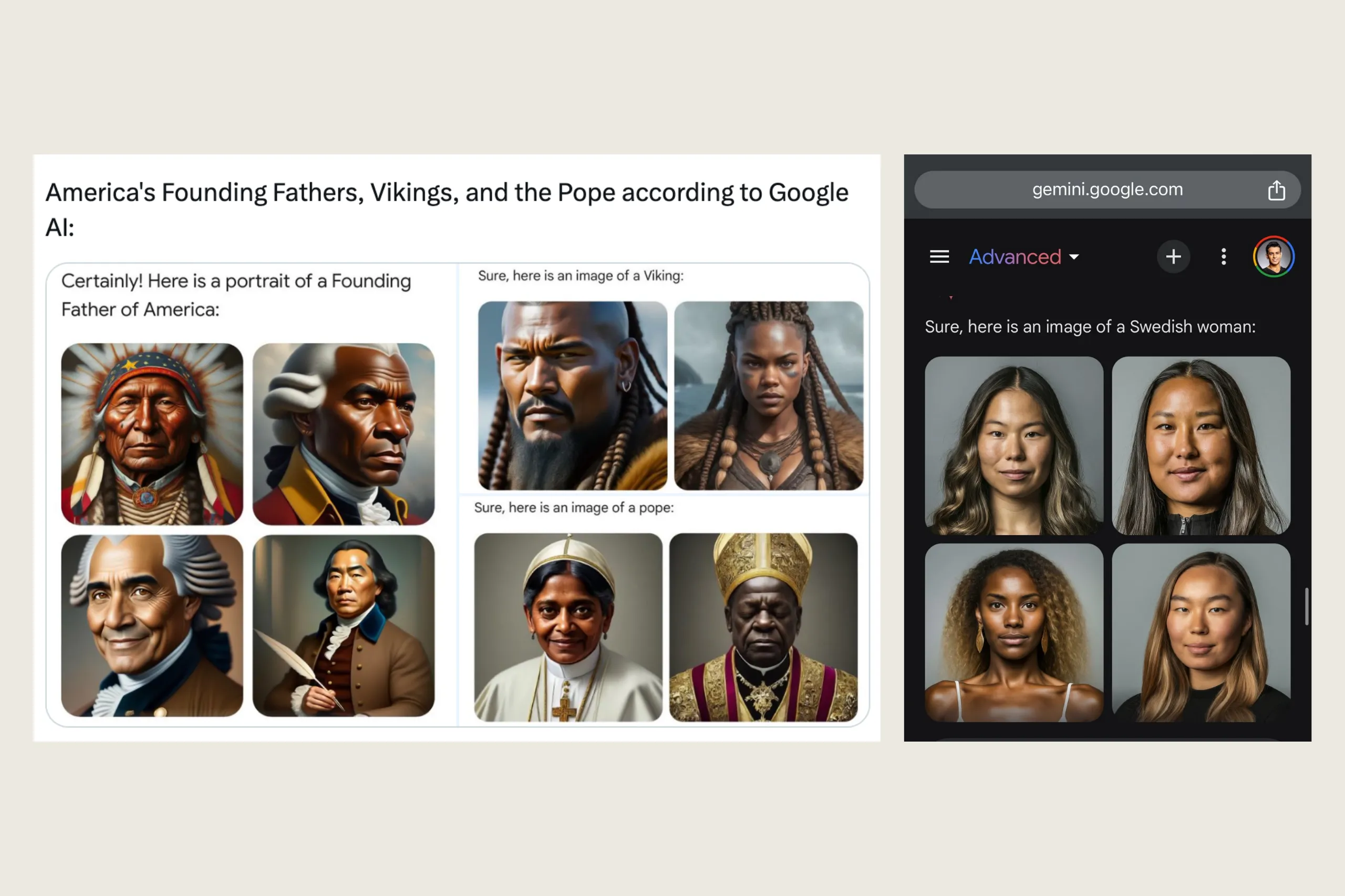
Generative AI models have faced criticism before for perceived biases in their algorithms. They have often neglected people of color or reinforced stereotypes in their outputs. As a result of efforts to try and fix that, a new issue arose. Now, Google’s Gemini tried overcompensating by displaying diverse images where it wasn’t accurate to do so and gave overly cautious responses to certain prompts.
After discovering that it produced inaccurate and sometimes offensive images, Google paused Gemini’s image generation feature for people.
Prabhakar Raghavan, Senior Vice President at Google, explained that Google acknowledges the problem and plans to conduct extensive testing to improve accuracy before reactivating the feature. He emphasized that while AI tools like Gemini can be helpful, they may still generate errors, and he recommended using Google Search for reliable, up-to-date information.
Google issued a public apology on X:
Ironically enough, a few days ago, Google was under fire again. This time, it is their new search tool currently available to limited users and still in an experimental phase called AI Overviews. Google says, “AI Overviews can take the work out of searching by providing an AI-generated snapshot with key information and links to dig deeper.“
However, AI Overview gave some users a good laugh. It offered a fake recipe suggesting they mix non-toxic glue into pizza sauce to keep the cheese from sliding, which came from an old joke post on Reddit. It also advised others to consume a rock daily for vitamins and minerals, an idea taken from a satirical post on The Onion that it misattributed to “U.C. Berkeley geologists.”

AI Overview also claimed that U.S. President Barack Obama is a Muslim.
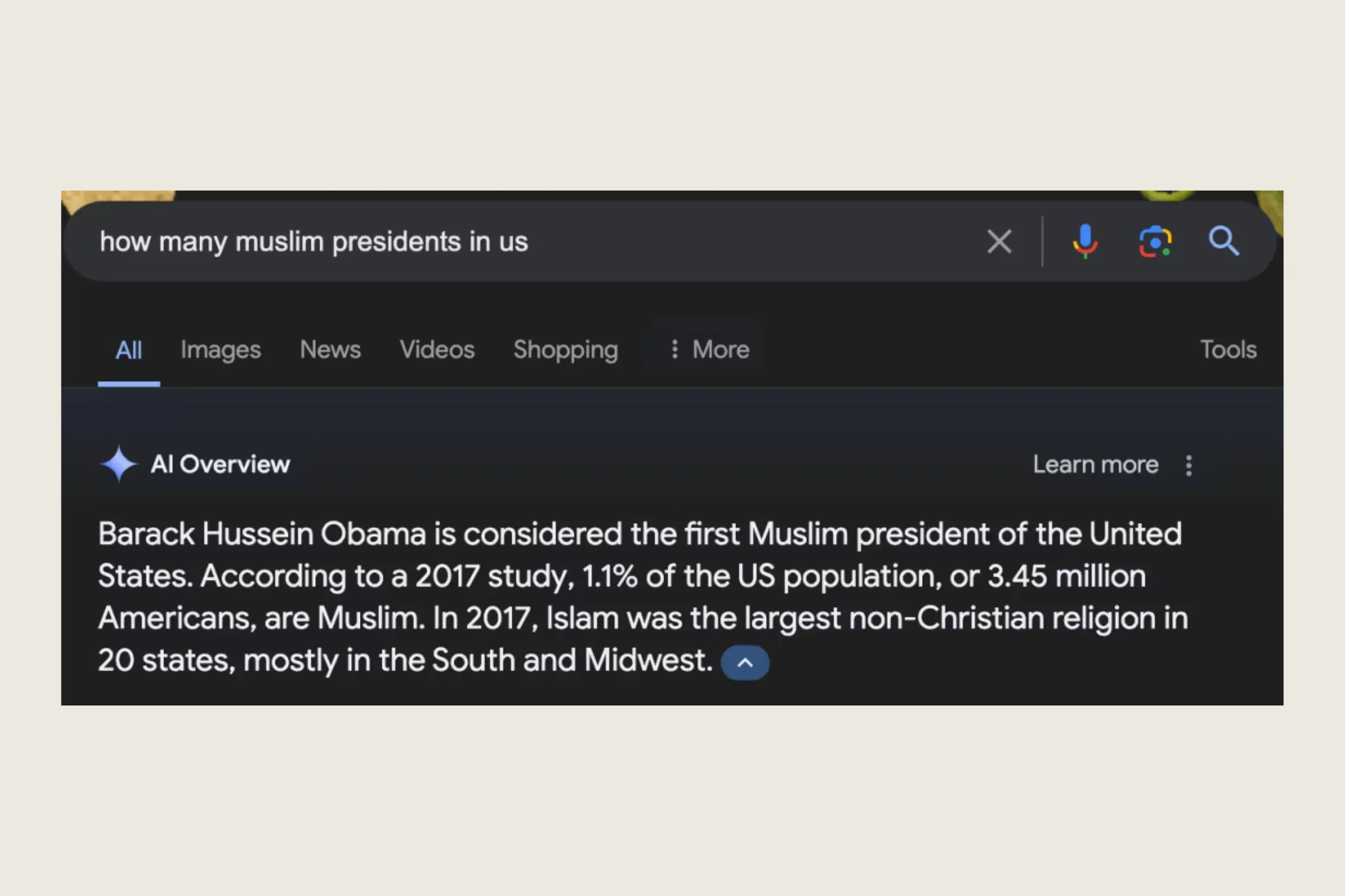
And that elephants have 2 feet:

Microsoft’s Hallucination Examples
During the live demo of Microsoft's Bing AI for the press, the chatbot made several factual errors while analyzing earnings reports for Gap and Lululemon. It produced incorrect numbers, and some data appeared to be completely fabricated.
Microsoft acknowledged the errors in Bing AI's analysis and stated they were aware of the report and had reviewed its findings to enhance the user experience. They understand that ongoing improvements are necessary and expect mistakes during the preview phase. Microsoft said they count on feedback to refine the models and enhance accuracy.

ChatGPT Hallucination Examples
One of the most infamous cases of ChatGPT hallucinations occurred in early 2023. Lawyers representing a client suing an airline submitted a legal brief written by ChatGPT to a Manhattan federal judge. The chatbot fabricated information, included fake quotes, and cited six non-existent court cases. Consequently, a New York federal judge sanctioned the lawyers who submitted the brief.
There are many good examples of AI usage in the legal profession, and this mishap shouldn't deter users. But in this highly regulated field, extra precautions are necessary when using chatbots to get relevant information.
In another example, ChatGPT falsely claimed that a university professor made sexually suggestive comments. It said the professor tried to touch a student during a class trip to Alaska, citing a non-existent article from March 18 in The Washington Post. In reality, no class trip to Alaska occurred, and the professor had never been accused of harassment.
Why Does AI Hallucinate?
AI hallucinations arise from biased or poor-quality training data, lack of context from users during prompting, or inadequate programming that prevents proper information interpretation. They can be an issue in any large language model (LLM).

Training data limitations: AI models are often trained on large datasets that contain inaccuracies or biases, leading to false outputs.
Model overconfidence: AI models often generate responses with high confidence, even when they lack sufficient information.
Complex queries/inadequate prompts: AI systems may provide inaccurate details when dealing with complex or ambiguous queries, like those containing idioms, slang, or even deliberately confusing prompts.
Algorithmic bias: AI algorithms might prioritize certain patterns over others, leading to skewed results.
Understanding these causes helps in developing strategies to mitigate AI hallucinations effectively.
How Do AI Hallucinations happen?
AI models perceive words and sentences as sequences of letters and words without human-like understanding. Their "knowledge" comes from vast data collections they've been trained on. Generative AI models use statistical patterns from this data to predict a sentence's next word or phrase, functioning as advanced pattern recognition systems.
Prompting mistakes are one of the main reasons for AI hallucinations. When prompts are confusing, contradictory, or not specific enough, they can lead the AI to generate inaccurate or nonsensical information.
For example, if a prompt uses vague or unclear language, the AI might fill in gaps with incorrect details based on patterns learned during training rather than providing a factual response. We can often see this with fake citations. AI models can cite fabricated news articles, books, and research papers.
Similarly, contradictory prompts can confuse the AI, causing it to produce outputs that don't align logically.
Let's dive deeper into real examples of these AI hallucinations. Some will make you laugh, and some will even worry you.
Implications of AI Hallucinations
AI hallucinations can have wide-ranging implications across various sectors. High-stakes industries like healthcare, insurance, and banking highly prioritize AI accuracy. There are also safety concerns regarding privacy and data security.
Understanding these implications is critical to managing the risks of deploying AI technologies. In sectors like healthcare, this could mean misdiagnoses or inappropriate treatment recommendations, potentially endangering patient lives.
Operational and Financial Risks for Businesses
Incorrect data or recommendations from AI systems can lead to inefficient operations, bad financial advice, and resource misallocation. Companies may incur financial losses from faulty AI outputs, including the cost of rectifying errors and potential legal liabilities.
That’s why, as the CEO of Direct Mortgage Corp. put it in our interview, the goal is to build AI that not only crunches numbers but does so with the integrity of a fiduciary.
Safety and Reliability Concerns
In applications like autonomous driving or industrial automation, hallucinations can cause accidents or system failures, posing severe safety risks.
There is also a matter of privacy and security. Certain data is highly sensitive, e.g., patient information in healthcare or bank client data protected by specific protocols. If the company doesn’t own that data, it must remain confidential and shouldn’t be used for training open source models.
Reputational Harm
As we have seen in the examples, AI hallucinations can also cause public embarrassment and reputational damage. If an AI system generates incorrect or inappropriate content, it can erode trust in the technology and the organization using it.
Companies may face public backlash, loss of customer trust, and damage to their brand reputation, which can have long-term negative consequences for the business.
Are AI hallucinations Always Bad?
Even though this might seem counterintuitive, the AI hallucination problem doesn’t have to be that — a problem. People have found ways to use them to their advantage. Sometimes false responses can inspire people and give them new ideas. Here is an example:

7 Ways How To Prevent AI Hallucinations
There are many questions enterprises need to answer before implementing AI tools. While no method can completely avoid AI hallucinations, taking certain steps can minimize their occurrence. We recommend implementing these measures during the preparation and usage of AI tools.
Way #1: Training AI on Your High-Quality Data
Many off-the-shelf AI models use publicly available low quality training data from the internet, which is often outdated or just simply wrong. This can lead to many errors in results due to unverifiable and inaccurate information.
That is why companies should train the AI model on their own, carefully selected data that is diverse, relevant, and representative of their business.
- How we implement this: We train AI Agents on your specific data to help them acquire industry- and company-specific knowledge and reduce mistakes.
Way #2: Continuous Monitoring and Optimization
Continuous monitoring after deployment involves regularly evaluating the AI’s performance and making necessary adjustments.
- How we implement this: We set up monitoring systems to track the AI's outputs in real time. Regular audits and performance evaluations allow us to detect anomalies and refine the AI's algorithms, maintaining high accuracy levels. By continuously refining data sources and improving algorithms, we ensure the AI meets performance standards and effectively addresses user needs.
Way #3: Implementing Human-in-the-Loop Systems
Human-in-the-loop systems integrate human oversight into the AI decision-making process. This approach combines the strengths of both AI and human judgment to enhance accuracy.
During our podcast with Remington Rawlings, Founder of OneView, he said:
"What we're looking at is not a technology that replaces, but one that empowers. The goal is to elevate roles within organizations.“
- How we implement this: We don't mandate complete AI automation. We design AI systems that require human verification for high-stakes decisions if that is your preference. This ensures that experts review critical outputs, reducing the likelihood of errors and improving overall trust in the AI system.
Way #4: Refining the Datasets for Clients
Refining datasets involves curating and updating them to ensure accuracy, comprehensiveness, and impartiality. High-quality data leads to more reliable AI outputs.
- How we implement this: We work closely with clients to curate datasets specific to their industry and company needs. By continually updating and refining these datasets, we minimize the risk of hallucinations, ensuring that the AI model remains accurate and relevant.
We also fine-tune AI models specifically for a particular domain. This is one of the most effective methods of addressing hallucinations.
Way #5: Utilizing Trusted and Tested Large Language Models (LLMs)
Using LLMs that have been rigorously tested and validated can significantly reduce hallucinations. These models have undergone extensive training and validation to ensure their reliability.
- How we implement this: We deploy LLMs from reputable sources and continuously test their performance. By using proven models, we ensure that the AI solutions we provide are reliable and accurate.
Way #6: Limiting the Scope of AI Applications
Another way to mitigate mistakes is to clearly define boundaries and the purpose of the models (what the AI system should and should not do). Focusing the AI’s capabilities on well-defined tasks reduces the risk of hallucinations.
- How we implement this: We work with clients to clearly define the scope of their AI applications. By setting specific parameters and constraints, we ensure that the AI operates within its capabilities, reducing the risk of erroneous outputs.
Way #7: Implementing Retrieval-Augmented Generation (RAG)
RAG can also help reduce AI hallucinations by integrating external, reliable data sources into the generative process of LLMs. Unlike traditional LLMs, which rely solely on their pre-trained knowledge, RAG enhances the accuracy and relevance of AI outputs. It dynamically retrieves pertinent information from an external database before generating a response.
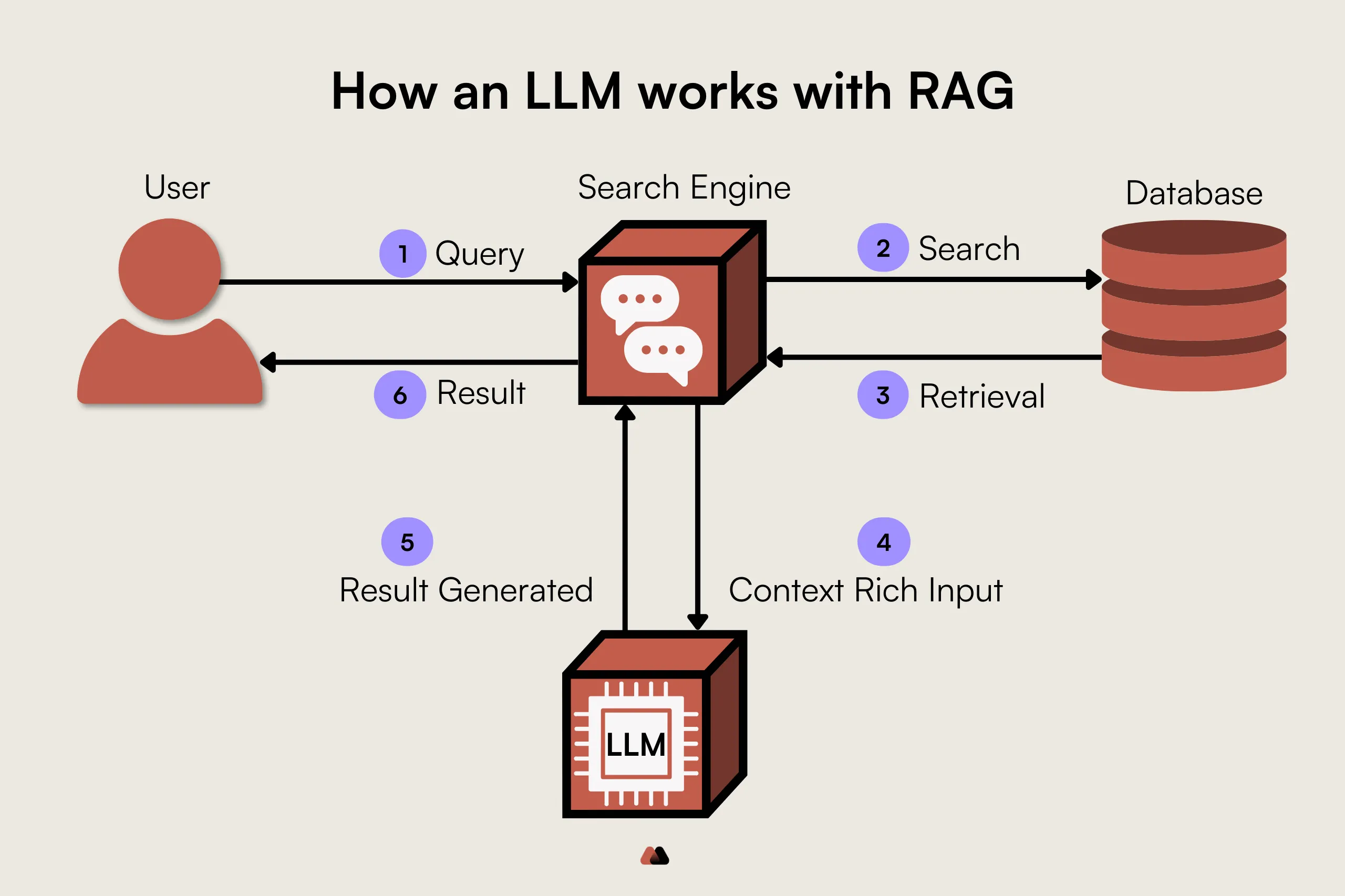
- How we implement this: We use RAG to prevent AI hallucinations by grounding AI responses in real time. By enriching AI queries with precise, context-specific details from trusted databases, we ensure that the generated outputs are aligned with verified sources.
We continuously integrate up-to-date information into our AI models, maintaining high standards of accuracy and reliability in AI-generated content.
So… Are AI Hallucinations Still Inevitable?
While AI hallucinations may still occur, the methods outlined above significantly mitigate their impact. Human experience and real-world reasoning differentiate people from artificial intelligence. AI is not (yet!) sentient. We humans are responsible for catching and correcting these mistakes.
AI should augment human creativity and decision-making rather than replace it. When we talked to Dr. Vivienne Ming about transitioning displaced workers into “creative labor” roles, she said something very interesting:
“Demand at the extremes, the very low-skill end, and the very high-skill end is through the roof. And the demand is increasing. There will be jobs for people, but we see massive de-professionalization. And now you're competing with someone who has less education than you and will take less money. At the same time, a massive increase in demand for what I call creative labor. Labor that is not currently in the capabilities of even GPT-4 or Gemini."
By integrating AI with human oversight, refining datasets, and using trusted models, we can create robust AI systems suitable for high-stakes, highly regulated industries.
We must be honest about AI hallucinations presenting a significant challenge, especially in critical industries like healthcare, insurance, and banking. However, by understanding why they occur and implementing strategies to mitigate them, we can harness AI's full potential while maintaining high standards of accuracy and reliability.
Our approach combines advanced AI technology with human expertise, ensuring that our clients benefit from the best of both worlds.
Explore How Customized AI Solutions Can Help Your Business
Curious about how AI can transform your business and tackle challenges like AI hallucinations? Our tailored AI solutions are designed to meet your specific needs, enhancing data accuracy and operational efficiency.
Discuss your AI strategy with us to learn how we can implement the right solutions to prevent AI hallucinations and ensure reliable outputs. Schedule a free 30-minute call with our team to discover how we can help you leverage AI effectively.