Routine tasks hinder productivity because employees can’t focus on higher-value tasks, leading to inefficiencies and increased operational costs.
Artificial intelligence (AI) tools like generative AI and predictive AI are viable solutions, but it isn’t easy to figure out which one to choose.
We’ll cover the core differences and various examples to help you make an informed decision best suited for your organization’s specific challenges.
Key Takeaways
- Generative AI excels at creating new content and automating tasks.
- Predictive AI focuses on analyzing data to forecast future events and make data-driven decisions.
- Different training techniques are used for generative AI and predictive AI to better adjust them for their purposes.
- Generative AI automates workflows and personalizes customer experiences, but requires careful data handling to avoid privacy issues. Predictive AI helps make informed decision-making but relies heavily on high-quality historical data.
- Generative AI and predictive AI can work together, as generative AI can leverage predictive capabilities to enhance its outputs.
- Prescriptive AI goes further than predictive AI by recommending the best action to take.
What is the Difference Between Generative AI and Predictive AI?

The difference between generative AI and predictive AI is that generative AI creates new content or data based on the input, while predictive AI analyzes existing data to identify patterns and trends used to forecast future outcomes.
For your business, this means generative AI is ideal for tasks like automating workflows, and predictive AI is best suited for making informed decisions and managing risks.
Difference #1: Function and Purpose
Generative AI is mainly used to create new data or content. It’s ideal if your business is looking for a solution to handle tasks that require originality and automation. This is because generative AI models are trained on large datasets. The model learns the underlying patterns and relationships within this data.
Meanwhile, predictive AI uses historical data, patterns, and trends to provide data-driven insights. As a result, businesses can make accurate predictions about future events, giving them a competitive advantage.
Difference #2: Training Techniques
As generative AI is usually trained on a much larger and more diverse dataset, it uses different machine learning methods compared to predictive AI. It typically uses techniques like generative adversarial networks (GANs) and variational autoencoders (VAEs) to better understand relationships in the large training dataset.
Predictive AI uses techniques that are better suited for making predictions. This includes techniques like:
- Supervised learning
- Linear regression
- Decision trees
These methods are specifically designed to analyze data, identify patterns, and forecast future outcomes based on the learned patterns.
Difference #3: Output
Generative AI produces original content, making it useful for applications like customer support. For example, Conversational AI is an AI Agent that analyzes unstructured documents to accurately answer customer and employee queries.
In healthcare, this could entail using unstructured data from healthcare records to handle queries from medical staff and patients. As a result, it makes key information more accessible and improves patient satisfaction.
Predictive AI usually provides outputs that are predictions or forecasts.
It can analyze patient history and current health data to predict future health events. For example, it can forecast the likelihood of a patient developing a chronic disease, so that healthcare providers can provide personalized treatment plans.
Generative AI vs. Predictive AI: Examples
To help you make the best choice for your business tasks, we’ll highlight some applications of both Predictive and Generative AI in healthcare, insurance, and finance.
Generative AI Examples
Generative AI can be used for:
- Healthcare: Generating personalized treatment plans and reports summarizing patient data, or drafting discharge instructions based on medical records.
- Insurance: Automating policy creation and renewal processes with pre-filled documents based on customer information.
- Finance: Generating custom financial reports or personalized investment recommendations for clients.
Predictive AI Examples
Some predictive AI applications include:
- Healthcare: Predicting patient readmission risks, identifying patients with a high chance of developing chronic diseases, or forecasting hospital resource needs.
- Insurance: Analyzing historical claims data to predict future claims and fraud, assessing risk profiles of new policy applicants, or personalizing insurance offerings.
- Finance: Forecasting credit defaults, predicting customer churn, or anticipating stock market trends.
Predictive AI vs. Generative AI: Advantages
Benefits of predictive AI include:
- Informed decision-making: Predictive AI uses past data like market trends to help businesses make informed decisions.
- Improved risk management: Banks, for example, can use large amounts of historical data to predict threats like identity theft and better prepare to prevent them.
- Personalized customer experience: Predicts customer behavior and preferences for tailored services, such as customizing policy options based on customer profiles.
- Resource Optimization: Businesses can better manage their resources based on predictions. Insurance companies can analyze historical claims data to forecast future claims.
Pros of generative AI include:
- Cost efficiency: Generative AI drastically lowers costs by automating routine tasks and minimizing the chance of human error.
- Improved productivity: Database AI, for example, uses your organization’s internal databases to answer customer and employee queries 20x faster.
- Enhanced content creation and report generation: AI Agents like Decision AI can generate reports that explain how they reached a decision, and Content AI helps businesses create on-brand content.
- Improved operational efficiency: Generative AI automates repetitive tasks like data entry so that employees can focus on higher-value tasks like strategic planning.
Predictive AI vs. Generative AI: Disadvantages
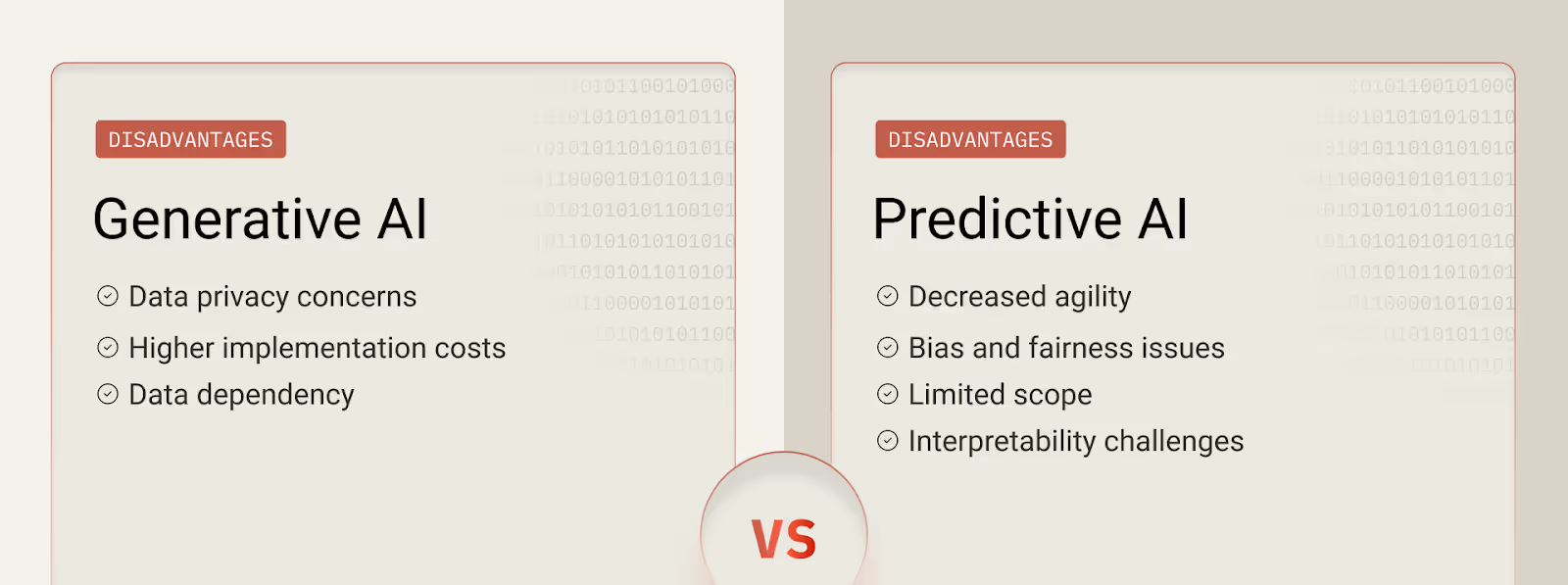
While predictive AI has its fair share of advantages, it also comes with a few drawbacks:
- Decreased agility: Focusing heavily on past data might make businesses less adaptable to sudden market shifts or disruptions.
- Bias and fairness: Predictive models can inherit biases present in historical data, leading to unfair outcomes in areas like loan approvals.
- Limited scope: Predictive AI is limited to forecasting based on past data and can’t generate new content or ideas, which limits its applications.
- Interpretability: Some predictive models, like deep learning, are difficult to interpret, so businesses have issues understanding how they came to a decision.
A few downsides to generative AI tools are:
- Data privacy: If sensitive data is used during the training process, it could be used in the outputs and lead to privacy breaches.
- Higher implementation costs: Although generative AI helps businesses save costs in the long term, the initial costs can be higher than with predictive AI solutions.
- Data Dependency: Accurate outputs require large volumes of high-quality training data, which might be limited in specific domains. Generative AI is also prone to hallucinations, especially in more complex models.
- Regulatory and Compliance Issues: Using generative AI in sensitive areas may involve navigating complex regulatory landscapes, especially concerning data privacy.
Can Generative AI Also Be Predictive?
Yes, Generative AI can also be predictive. While generative AI and predictive AI are distinct in their primary functions (generating new data and predicting future outcomes), there’s an overlap in their capabilities. Generative AI models usually make use of predictive elements to boost their performance and utility.
👉 So-called hybrid models combine generative models with predictive techniques, or vice versa. They can both generate new data and make predictions based on existing data. Hybrid models are extremely popular in highly regulated, high-stakes industries. We use them in almost all client projects.
How Generative AI Incorporates Predictive Capabilities
Generative AI can be combined with predictive capabilities for different healthcare, insurance, and finance applications.
- In healthcare, generative AI models can predict and generate medical notes, patient histories, and treatment plans based on the context of previous entries. When a healthcare professional inputs the initial patient symptoms, the model predicts and suggests the most relevant diagnostic tests and treatment options.
- In insurance, generative AI can enhance underwriting by predicting risk levels and generating policy recommendations. When underwriters input applicant data like demographics and previous claims, the model predicts the chance of future claims and suggests appropriate coverage plans. This lets businesses decrease risk.
Generative AI vs. Predictive AI: Use Cases
We’ve covered some general examples of how generative AI and predictive AI have been used in healthcare, insurance, and finance settings, but we’ll cover specific use cases to help you better understand the differences between the two.
Generative AI: Use Case #1
Doctors used GPT-4 to simplify complex surgical consent forms, reducing them from three pages to a single easy-to-read page. This improved patient understanding and comfort. The word count of consent forms was reduced by 25%, and the health system is now applying this process to other medical documentation.
Generative AI: Use Case #2
Insurers implemented generative AI in their software testing lifecycle to optimize regression testing and improve test coverage. This led to expected savings of 40% in efforts, 35% optimized regression testing, and 32% overall quality assurance cost savings through efficiencies.
Predictive AI: Use Case #1
One of our clients uses predictive AI to automate healthcare documentation, drastically reducing administrative burden. More specifically, they automated the labeling of medical notes with exceptional accuracy, allowing clinicians to focus more on delivering high-quality patient care.
Predictive AI: Use Case #2
Another use case of predictive AI is mortgage processing. Extracting information from complex tax forms manually is expensive and highly error-prone. Predictive AI automated this process with near-perfect accuracy and carried out document extraction 10x faster than manual methods, allowing for faster data processing.
Other Types of AI: Prescriptive AI

Prescriptive AI goes a step further than predictive AI. Instead of only making predictions based on past data, prescriptive AI recommends the best course of action.
Key Characteristics of Prescriptive AI:
- Action-oriented: Unlike descriptive analytics, which describes what has happened, and predictive analytics, which forecasts what will happen, prescriptive analytics determines the best steps to take in response to those insights. It aims to answer the question, "What should we do?"
- Complex algorithms: Prescriptive AI relies on more advanced algorithms such as decision trees to provide actionable recommendations. These models analyze data to identify optimal decisions under given constraints.
- Integration with predictive models: Prescriptive AI often integrates with predictive models to improve its recommendations. For example, it uses predictive insights about future demand to suggest how much stock to order or which marketing strategies to implement.
- Real-time decision making: It enables real-time decision-making by continuously analyzing data and updating recommendations as new information becomes available. This dynamic approach helps businesses adapt quickly to changing conditions.
Examples of Prescriptive AI in Action
In healthcare, predictive AI could determine which disease a patient has. Prescriptive AI would then go further by recommending personalized treatment plans for patients based on:
- Their medical history
- Current health conditions
- Predictive models of disease progression
Predictive AI models in finance assess the credit risk of loan applicants. By analyzing historical data on loan repayments and applicant financial histories, these models can predict the chance of default for new applicants, so banks can make informed lending decisions.
A prescriptive AI system would take the next step by recommending personalized repayment plans or interventions for high-risk borrowers.
For example, it could:
- Suggest restructuring loans
- Provide actionable financial advice
- Implement automated reminders for payments
This helps reduce default rates but also supports borrowers in managing their debts more effectively.
Which One Should You Choose?
It’s best to start by assessing your business's challenges. If you notice that you need a solution that will automate repetitive tasks like data extraction or produce original outputs, generative AI would be the right option.
Otherwise, you might be having issues with making informed decisions and predicting future trends. In this case, predictive AI would be an appropriate solution.
While both generative and predictive AI offer unique advantages, we believe that a hybrid model allows businesses to take advantage of both approaches, providing the most comprehensive solution. This combined approach allows organizations to use generative AI's adaptive capabilities while benefiting from predictive AI's forecasting power.
To learn how your business can implement a hybrid model to automate workflows and boost productivity, schedule a 30-minute call with our experts and see a live demo.