This is a summary of an episode of Pioneers, an educational podcast on AI led by our founder. Join 2,000+ business leaders and AI enthusiasts and be the first to know when new episodes go live. Subscribe to our newsletter here.
TLDR:
- Companies should tie artificial intelligence usage clearly to business value like revenue or customer retention
- Don't assume another company's AI success will directly translate to your own
- Establish rigorous risk management for AI systems
- Frame artificial intelligence as enhancing human productivity rather than replacing jobs
While artificial intelligence is gaining more mainstream appeal thanks to chatbots and generative AI like ChatGPT, other advances in machine learning and language models are opening new possibilities for enterprises to amplify human potential.
Most companies can't afford to ignore AI — but you also can't just jump in without a strategy. Successfully leveraging artificial intelligence requires careful planning and responsible adoption.
We recently sat down with Wayne Butterfield, Partner and Global Lead of Intelligent Automation at ISG, to walk through his AI adoption playbook and discover how companies can:
- Pinpoint the right opportunities for workflow augmentation in your business
- Avoid AI pitfalls that have killed ROI for other companies
- Build an adoption roadmap that brings human-AI collaboration to the forefront of your industry
Check out the full interview here:
Meet Wayne - Automation Pioneer
Wayne Butterfield, currently a Partner and the Global Lead of Intelligent Automation at ISG, shares his background and experience on how his passion for technology helped him learn how to use technology to solve business challenges using automation.
With early experience in dealing with chatbots, digital customer service, and automation, Wayne helped replicate and use his knowledge to help ISG set up automation practices.
With over 15 years of experience of being at the forefront of AI (more recently Gen AI) and intelligent automation, he’s been pioneering solutions and guiding businesses through digital transformation.
Defining Automation, Machine Learning, and Generative AI
"AI has become a bit of an umbrella buzzword that encompasses a lot of technologies"
Developing an effective AI adoption strategy helps companies segment AI capabilities into those that automate tasks by mimicking repetitive, rules-based human workflows and those that enhance decision-making by detecting patterns and predictions.
This includes:
- Automation: One example is robotic process automation (RPA), which uses software "robots" to emulate human actions and perform repetitive, rules-based tasks using structured data. RPA excels at high-volume, mundane workflows like transferring data between systems.
- Machine learning: AI can leverage machine learning algorithms that statistically "learn" from data without explicit programming. Whereas first-generation RPA has limits in dynamic environments, modern automation leverages AI and machine learning to adapt, providing a scalable path to increased efficiency.
- Generative AI: This category of AI can dynamically generate brand-new content, outputs, and other predictions. The most revolutionary generative AI approach uses natural language models like OpenAI's ChatGPT that can engage conversationally.
Wayne notes generative AI is opening "this whole new Pandora's box of what can now be deemed as automatable." Unlike RPA's ceilings, "the complexity of the work that we are completing deemed appropriate for automation keeps increasing."
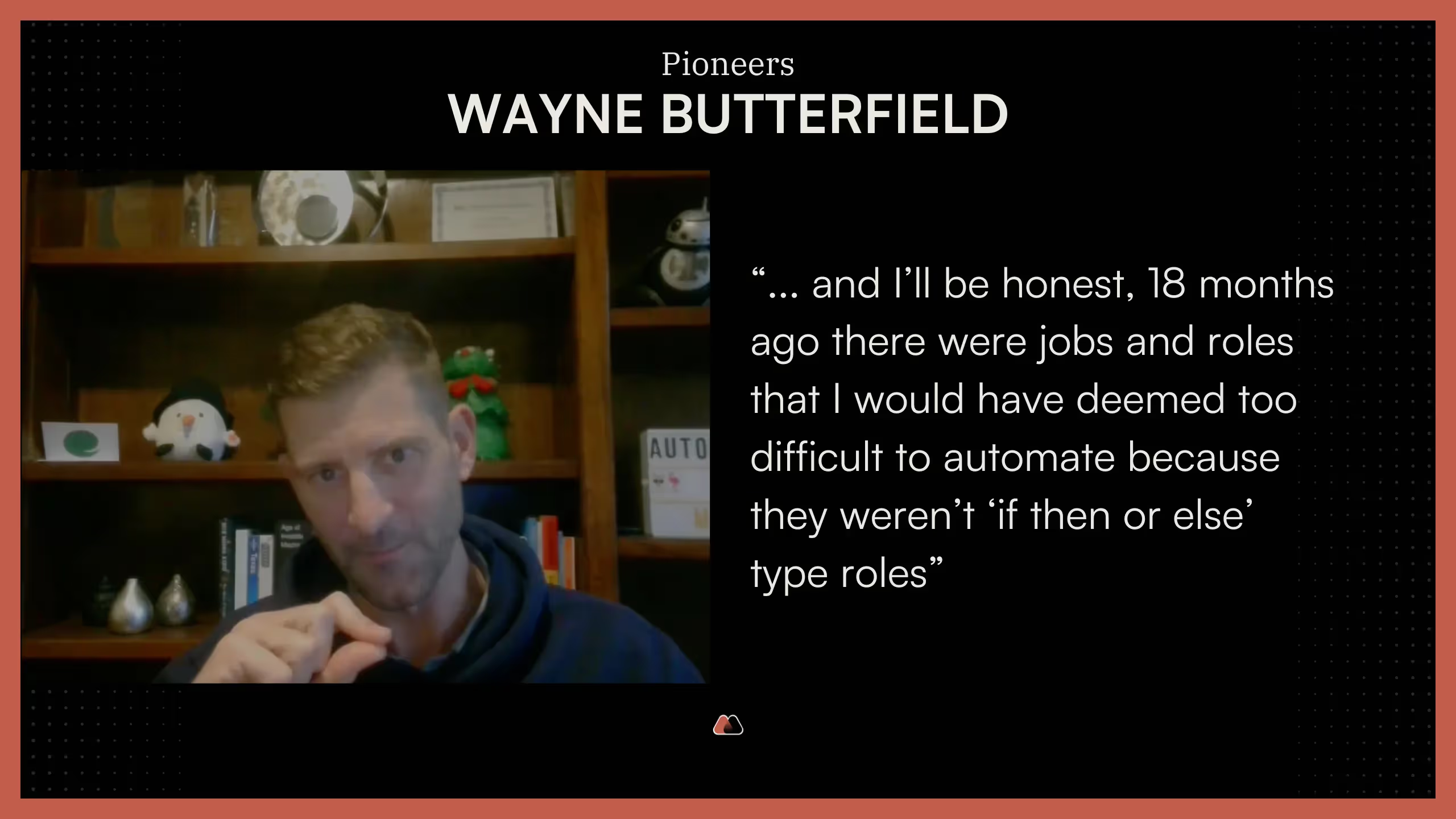
Artificial Intelligence Adoption: Identify Your AI Automation and Augmentation Opportunities in 6 Steps
The key is to tailor AI’s specific applications to your specific organization and priorities right now.
Wayne lays out six key steps to identifying gaps in your company’s technology capabilities and business context that artificial intelligence could potentially fill.
Based on Waye’s experience, he also adds that many companies aren’t ready to let AI make decisions for them.
Both Ankur and Wayne agree that recommendations (especially in the early stages) make the best approach to AI adoption, and as Ankur would describe the process of using AI after implementation: trust but verify.
1. Document Core Processes First
"If you can't explain it, if you can't document it, then you can't automate it,” says Wayne. Without a properly documented process, AI effectiveness can deteriorate.
That’s why it’s important to review existing process documentation to identify automation potentials and gaps. Enlist process experts across company functions to capture tribal knowledge into playbooks.
2. Analyze Your Current Workflow
From an operational perspective, Wayne advises examining workflows for:
- Structured vs. unstructured inputs
- Static vs. dynamic environments
- Clear vs. ambiguous decision rules
"Hand work is when we're using our mouse, making a few clicks, pointing at some stuff, and doing quite transactional work," Wayne explains, the sweet spot for automation.
Head work is more complex, cognitive efforts that may be harder to automate. Consider where automation can not only save costs but also unlock human creativity and judgment.
3. Prioritize the Processes for Machine Learning Automation
Machine learning models need large, clean datasets to train on. Zero in on high-volume, structured data processes where patterns can be learned, such as transaction categorization, predictive maintenance, and customer lifetime value forecasting.
4. Shift Repetitive Tasks or “Hand Work” to Bots
Mundane, repetitive workflows remain an ideal target for automation like RPA, despite its limits. Many companies can find value in using this category of AI in IT, customer operations, and back offices.
5. Review Potential Roles for Generative AI
Rather than only looking at processes, analyze roles augmented with AI-generated insights, recommendations, and drafts. Generative AI can suit various content creation tasks like chatbots, sales proposals, and reporting. Apply machine learning predictions to boost human decisions everywhere, from recruiting to new product forecasting.
6. Think Big Picture
Don't limit your AI visions to minor optimizations — reimagine overhauled workflows with humans empowered by integrated automation. For example, your front-line staff could have on-demand AI advisors while data analysts receive automated data preparation and reporting.
The key to impact rests in AI augmenting rather than replacing people — in other words, "removal of stuff that is the least enjoyable part of your business,” Wayne says.
He advocates handling mundane tasks to free up talent for higher judgment.
"Let's take the heavy lifting out of any process we want to complete, but let's still put our eyes on any form of output.” - Wayne Butterfield
Avoiding the Common AI Adoption Pitfalls
With the right strategic plan, artificial intelligence can drive tremendous value. However, it’s also essential to know the common obstacles preventing companies from successfully leveraging artificial intelligence.
Here are some common pitfalls Wanye has seen — and you can avoid them:
Building Over Buying
With growing artificial intelligence adoption, executives face a decision around in-house capability development.
Wayne argues few companies can mimic the end-to-end innovation and productization of AI/ML accomplished by the likes of Amazon, Google, and Microsoft.
For most organizations, buying AI accelerators helps focus resources on domain expertise and data while leveraging technology partners.
Link AI to Business Value
As with any technology, AI should tie directly to business priorities and required outcomes. Artificial intelligence won't win over stakeholders on its own.
Instead, lead with the use case value — such as customer retention or revenue growth — when presenting AI plans and progress.
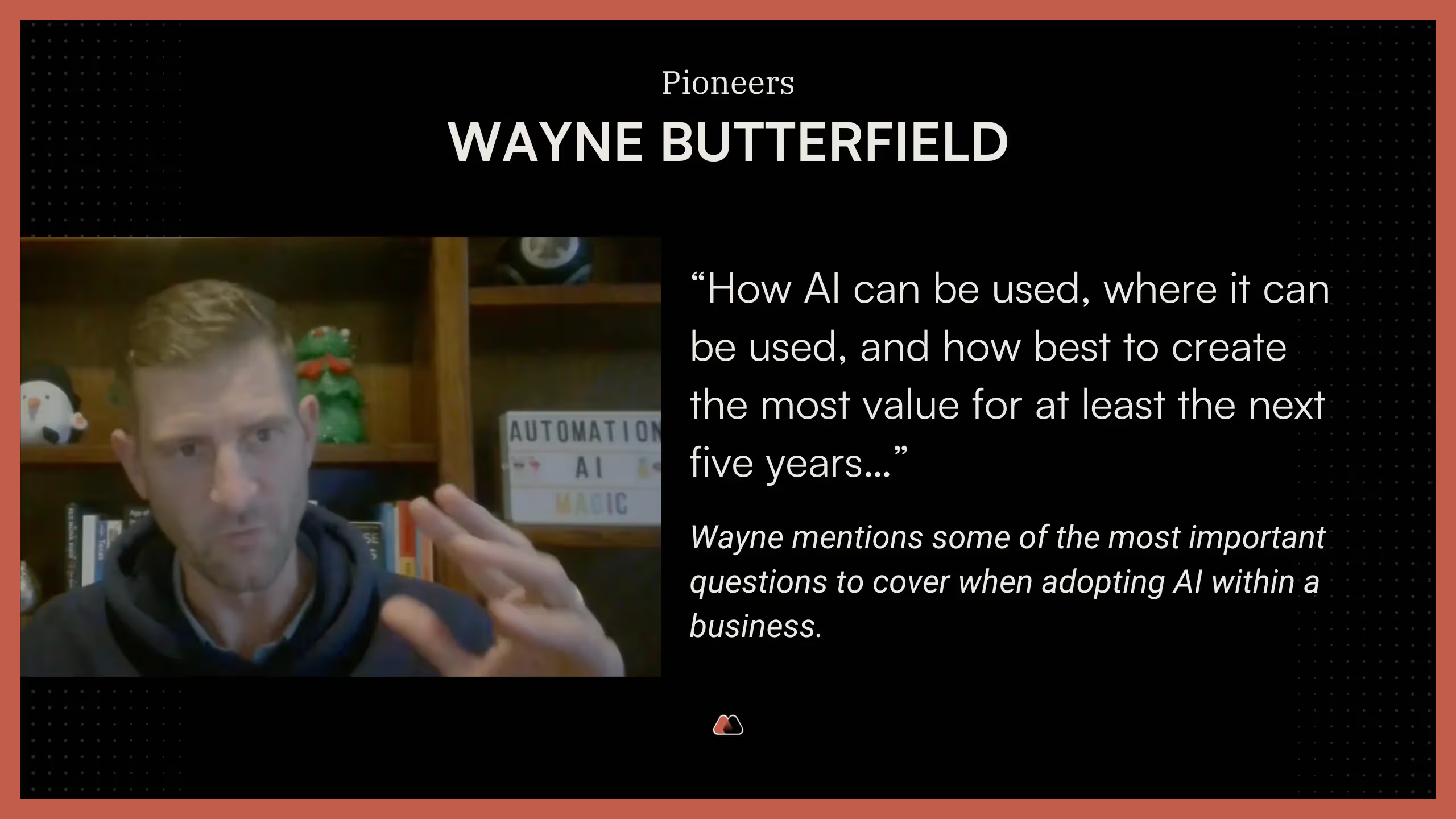
Assuming One Size Fits All
Repeating competitors' success stories won't cut it, says Wayne.
"Just because it works elsewhere doesn't necessarily mean it's going to work everywhere … Often it's not the technology's fault, it's your internal environment." - Wayne Butterfield
Think about how AI’s use cases apply to your organization's unique systems, data, and workflows.
Documentation Before Automation
Since artificial intelligence learns from data patterns, attempting automation without documentation is futile. Refine documentation first for transparency on responsibilities, decisions, and judgments made.
Not Fully Understanding the Risks
Like any business adoption, you should subject AI solutions to rigorous risk management.
It’s vital to establish human guardrails on AI augmentation to avoid fully autonomous decisions.
One way to do this is by assigning liability for AI outcomes to specific senior positions rather than absolving systems.
The Way Forward: Let AI Enhance Human Potential, Not Replace It
Wayne envisions an "autonomous enterprise of the future" with "workforce productivity through leveraging capability that works with them seamlessly rather than replaces people."
AI can relieve people of repetitive tasks like data searches, letting them concentrate on judgment, relationships, and critical thinking. Technicians can better diagnose problems informed by predictive recommendations. Marketers gain the capacity to drive strategy instead of cranking out content.
The augmentation paradigm also keeps accountability and ethics central by keeping a human in the loop for oversight. The long-term technical potential remains boundless.
"Every time we have this umbrella term ‘automation’ and it nitpicks at certain aspects of work," Wayne notes, "the tools keep changing, the capabilities of those tools keep improving, and suddenly the complexity of the work that we are completing that is deemed appropriate for automation keeps increasing."