This is a summary of an episode of Pioneers, an educational podcast on AI led by our founder. Join 2,000+ business leaders and AI enthusiasts and be the first to know when new episodes go live. Subscribe to our newsletter here.
TLDR:
- The concept of “big data” has evolved from focusing on volume to emphasizing data connectivity, accessibility, and usability with a shift toward cloud-based data approaches.
- Implementing AI and data solutions in large organizations requires a pragmatic approach that focuses on solving problems and showing value in short cycles.
- Successful implementation of data and AI solutions demands multidisciplinary teams and a need for talent that can bridge old and new technologies.
- Generative AI adoption in large organizations is likely to be rapid, but it requires end-to-end process changes.
- There’s a current lack of mature tooling for monitoring, managing, and maintaining Gen AI in production environments.
Before we dive into the key takeaways from this episode, be sure to catch the full episode here:
Meet Shivaji — Expert Chief Data Officer With AI Implementation Expertise
Shivaji Dasgupta started by coding and doing data analysis, and after getting the PhD, transitioned to management consulting and digital strategy in companies like McKinsey.
After leaving McKinsey, Shivaji was involved in data transformation initiatives in large banking organizations, where he gained experience implementing AI use cases, including generative AI.
In our podcast, Shivaji emphasizes a pragmatic approach to technology implementation and the importance of balancing new technologies with existing infrastructure in large enterprises.
Evolution of Data Management
“The concept of big data as it was 10 years ago does not really resonate anymore because of the technology which is already available and which can help you navigate data without actually making it big data.” — Shivaji Dasgupta
Data management has evolved significantly over the past decade, transitioning from a focus on “big data” characterized by large-scale storage solutions. Shivaji mentions how there’s been a step from large-scale storage solutions like Hadoop to more sophisticated approaches.
The new approach is centered on data connectivity and accessibility, where the initial goal was to handle vast volumes of data with on-premise systems.

Modern data management now prioritizes the seamless integration of data across various platforms, which ensures that the right data is accessible to the right users with appropriate security measures.
The focus is also shifting from centralized data repositories to a more distributed approach. Nowadays, data is managed more in context-specific platforms, which improves efficiency and scalability in analytics and decision-making processes.
Challenges Implementing AI and Data Solutions
When it comes to implementing AI and data solutions, Shivaji says the most common challenges include:
- Data accessibility
- Scalability of AI models
- Integration with legacy systems
- Proof of concept (PoC) issues
- Security and compliance
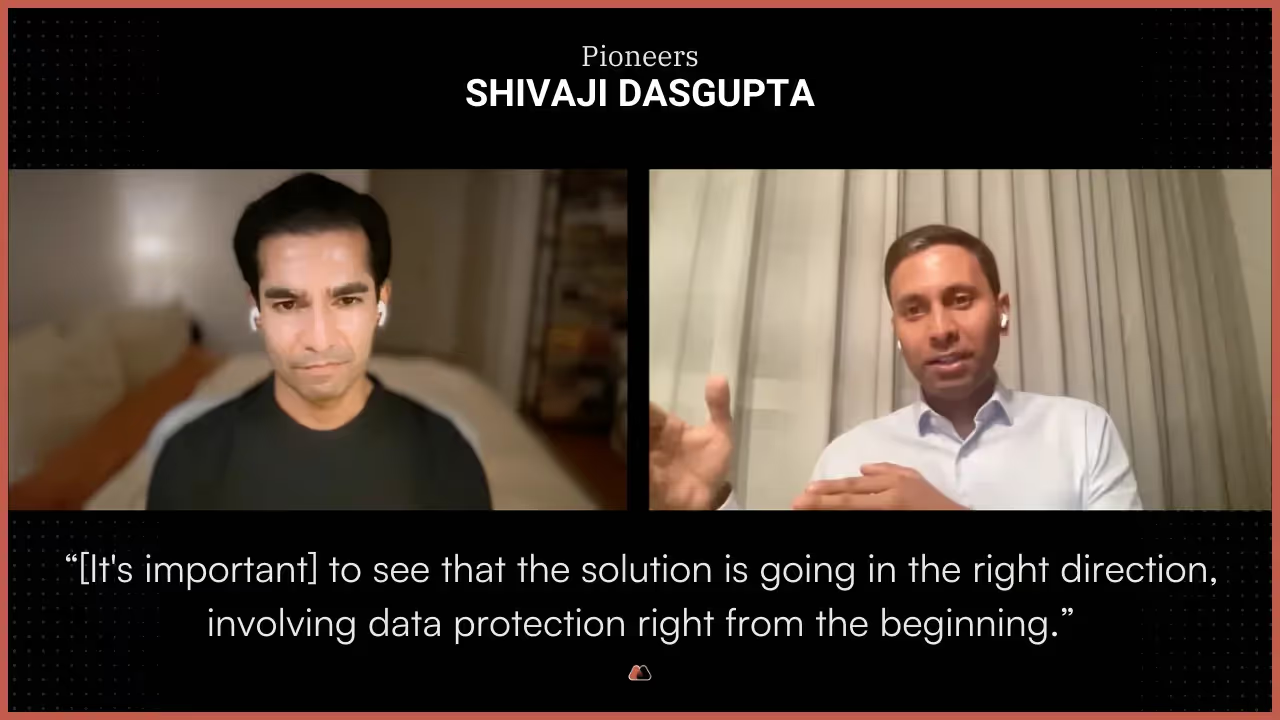
Shivaji mentions one of the key challenges is data accessibility because the right data needs to be available to the right users while maintaining strict security in regulated industries like banking.
Moving AI models from experimentation to production is another challenge that requires careful planning to ensure scalability and integration with existing systems.
Integration of new technologies comes with its own challenges, where integration of generative AI with legacy systems poses significant difficulties.
“We work with customers that are very large, complex, financial organizations, and it’s hard enough for them to find the relevant data for a proof of concept.” — Ankur Patel
Generative AI in Enterprise and Pragmatic Approach to Digital Transformation
In the context of enterprises, generative AI represents a powerful tool for enhancing and automating processes. It can revolutionize tasks like loan origination and customer interaction by automating decision-making processes and generating insights.
However, integrating gen AI into existing systems can be complex, especially due to challenges with legacy systems and the need for robust APIs and connectivity solutions.
Shivaji emphasizes the importance of pragmatism in deploying these technologies, advocating for a focus on practical and incremental improvements rather than large-scale disruptive changes.
Such an approach helps mitigate risks associated with generative AI, like operational disruption and data privacy concerns.
Data Connectivity and Integration
Data connection and integration are pivotal for transforming and enhancing the decision-making processes. Shivaji highlights the evolution from traditional big-data approaches to modern methods that emphasize data flow, accessibility, and quality.
He also mentions that the shift towards federated data and cloud-based analytics allows organizations to manage data more efficiently without centralizing it. However, integration of these modern solutions into existing systems presents earlier-mentioned challenges.
Shivaji’s pragmatic approach helps effectively integrate solutions into workflows by addressing complexities and ensuring that data integration efforts yield tangible benefits without significant operational risks.
“To get it there to make it work really well, it does require enough testing and enough human intervention. We should not discount that there is not at least still no generative AI out of the box that we can just put in and use.” — Shivaji Dasgupta
Team Composition and Skills
Building effective teams for data projects involves a multidisciplinary approach to tackle the complexities of modern data solutions. Shivaji emphasizes the need for diverse expertise, including product managers, operations, risk, compliance, IT, and data specialists.
Such a blend ensures that all aspects of data projects, from technical to regulatory, are addressed comprehensively. He points out that traditional siloed roles, such as separate chief data officers and chief information officers, are becoming less effective.
Instead, a product-driven organization with integrated responsibilities fosters better collaboration and innovation.

Shivaji’s earlier mentioned pragmatic approach also advocates for pragmatic team structures that focus on iterative development and practical solutions rather than aiming for perfect plans from the outset.
Such an approach enhances the implementation of data initiatives and aligns them closely with business objectives, which ensures new technologies are seamlessly integrated into everyday operations.
Regulatory and Ethical Considerations
Shivaji Dasgupta underscores the importance of transparency, explainability, and compliance with emerging regulations like the AI Act in Europe.
Ensuring data privacy, proper governance, and accountability are paramount, especially in industries with high regulatory scrutiny. Dasgupta emphasizes the need for robust frameworks to measure and monitor AI models, including clear KPIs for transparency and explainability.
A human-in-the-loop approach is ideal for mitigating risks associated with automated decision-making and ensuring that AI outputs are reliable and ethical. Such a strategy helps organizations navigate the complex regulatory landscape.
Shivaji mentions that relying on such strategies helps integrate new technologies in a way that maintains trust and compliance while leveraging the benefits of AI for enhanced operational efficiency.